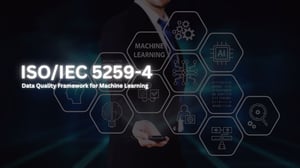
ISO-IEC 5259-4:2024
A standard for data quality in machine learning (ML)
Explore the world of ISO/IEC 5259-4, a pivotal standard that ensures data quality in analytics and machine learning. Discover how it enhances model performance, increases stakeholder trust, and supports regulatory compliance across industries.
Discover how ISO-IEC 38507 empowers organizations to govern AI systems responsibly. Learn about its framework for ethical AI use, risk management, compliance, and innovation support, and explore the key benefits it brings to organizations of all sizes and sectors.
ISO/IEC 5259-4 provides a comprehensive framework for ensuring data quality in training and evaluation processes for analytics and machine learning (ML). This standard establishes universal organizational approaches applicable to any organization regardless of type, size, or sector.
What is ISO/IEC 5259-4?
ISO/IEC 5259-4 is an international standard that establishes a data quality process framework for analytics and machine learning. Released in July 2024, it provides guidance on ensuring data quality across various ML methodologies, including supervised learning, unsupervised learning, semi-supervised learning, reinforcement learning, and analytics.
The standard addresses the entire data lifecycle, covering acquisition, composition, preparation, labeling, evaluation, and use. By focusing on organizational approaches rather than specific technologies, ISO/IEC 5259-4 remains adaptable to diverse organizational needs without prescribing particular services, platforms, or tools.
Key Components of the ISO/IEC 5259-4 Framework

Supervised Machine Learning
The standard emphasizes best practices for labeling data used to train ML systems. It provides common organizational approaches for training data labeling, ensuring consistency and accuracy in the supervised learning process. This is particularly important as labeled data forms the foundation upon which supervised ML models learn to make predictions.
Unsupervised Machine Learning
For applications without labeled data, ISO/IEC 5259-4 outlines processes to ensure data integrity. This includes methods for validating data quality when working with clustering, dimensionality reduction, and other unsupervised techniques where the system must identify patterns without explicit guidance.
Semi-supervised Machine Learning
The standard addresses the unique challenges of combining labeled and unlabeled data effectively. It provides frameworks for maintaining data quality when only a portion of the dataset contains labels, helping organizations leverage larger datasets while maintaining quality standards.
Reinforcement Learning
ISO/IEC 5259-4 includes specific guidance for managing data derived from interaction-driven methods. This encompasses approaches for ensuring quality in environments where data is generated through agent interactions, addressing the unique challenges of reinforcement learning data collection and evaluation.
Analytics Applications
Beyond machine learning, the standard enhances data quality for broader analytical applications. This ensures that organizations can maintain consistent quality standards across their entire data ecosystem, not just in ML-specific contexts.
Implementation and Global Adoption in 2025
Since its publication in July 2024, ISO/IEC 5259-4 has gained significant traction globally. In February 2025, the European Committee for Standardization (CEN) and the European Committee for Electrotechnical Standardization (CENELEC) adopted it as EN ISO/IEC 5259-4:2025, making it a European standard as well. This adoption has accelerated its implementation across European organizations seeking to enhance their ML data quality processes.
The standard was jointly proposed by iFLYTEK and the China Electronics Standardization Institute, marking China's first international standard in the field of AI data quality. This collaboration highlights the global nature of data quality challenges and the importance of international cooperation in addressing them.
According to recent developments, ISO/IEC 5259-4 is increasingly being integrated with other standards in the ISO/IEC 5259 series, particularly with ISO/IEC 5259-5:2025, which focuses on data quality governance frameworks. This integration provides organizations with a comprehensive approach to data quality that spans both technical processes and governance structures.
Benefits of Implementing ISO/IEC 5259-4

Organizations implementing ISO/IEC 5259-4 can realize numerous benefits:
Enhanced Model Performance and Reliability
By establishing standardized approaches to data quality, organizations can reduce errors and biases in their training and evaluation data. This leads to machine learning models that perform more reliably and accurately, delivering consistent results across applications.
Increased Stakeholder Trust
Improved data quality enhances the effectiveness of analytics and ML systems while building trust with stakeholders. By demonstrating a commitment to responsible and transparent data practices, organizations can strengthen relationships with customers, partners, and regulatory bodies.
Streamlined Workflows and Resource Efficiency
The standard helps organizations streamline their data preparation and evaluation workflows, reducing inefficiencies and saving valuable time and resources. This is particularly important as data preparation typically consumes 60-80% of data scientists' time in ML projects.
Cross-Industry Collaboration
The guidance provided by ISO/IEC 5259-4 is adaptable to diverse organizational contexts, making it easier for teams across industries to collaborate and maintain consistent quality standards. This facilitates knowledge sharing and best practice adoption across sectors.
Regulatory Compliance and Innovation Leadership
Compliance with ISO/IEC 5259-4 positions organizations as leaders in data governance and innovation. It helps them meet emerging regulatory requirements, avoid costly rework, and ensure long-term scalability and success in their AI initiatives.
Integration with the EU AI Act and Global Regulations
The implementation of ISO/IEC 5259-4 has become increasingly important in the context of the EU AI Act, which came into effect in 2024. Organizations seeking to comply with the Act's requirements for high-risk AI systems can leverage ISO/IEC 5259-4 as part of their compliance strategy.
The standard aligns with the EU AI Act's emphasis on data quality and governance, particularly in addressing requirements related to data preparation, management, and quality assurance. By implementing ISO/IEC 5259-4, organizations can demonstrate their commitment to responsible AI development and use.
Beyond Europe, ISO/IEC 5259-4 is being referenced in global AI regulations and frameworks worldwide. Its universal applicability makes it a valuable tool for organizations navigating the complex landscape of international AI governance.
Relationship with Other Standards
ISO/IEC 5259-4 is part of a broader ecosystem of AI standards that collectively provide a comprehensive framework for responsible AI development and deployment:
- ISO/IEC 5259-1: Defines the data life cycle model for analytics and ML
- ISO/IEC 5259-2: Focuses on data quality measures and metrics
- ISO/IEC 5259-3: Addresses data quality management requirements and guidelines
- ISO/IEC 5259-5: Provides a data quality governance framework
Additionally, ISO/IEC 5259-4 complements other AI standards such as ISO/IEC 42001, which focuses on AI management systems, and ISO/IEC 23894, which addresses risk management in AI systems.
Implementation Challenges and Best Practices
While ISO/IEC 5259-4 provides a robust framework for data quality, organizations may face challenges in implementation. Common challenges include:
Organizational Alignment
Ensuring alignment across different departments and teams can be challenging. Organizations should establish clear roles and responsibilities for data quality management, with executive sponsorship to drive adoption.
Technical Infrastructure
Implementing the standard may require updates to existing data infrastructure and tools. Organizations should assess their current capabilities and develop a roadmap for addressing gaps.
Skills and Expertise
Many organizations lack the specialized skills needed for effective data quality management in ML contexts. Investing in training and development or partnering with experts can help bridge this gap.
Best Practices for Successful Implementation
To maximize the benefits of ISO/IEC 5259-4, organizations should consider the following best practices:
- Start with a pilot project: Begin with a well-defined, manageable project to demonstrate value and learn from the implementation experience.
- Integrate with existing processes: Align ISO/IEC 5259-4 implementation with existing data governance and quality management processes to avoid duplication and ensure consistency.
- Measure and monitor progress: Establish key performance indicators (KPIs) to track the impact of improved data quality on ML outcomes and business value.
- Foster a data quality culture: Promote awareness and understanding of data quality principles across the organization, emphasizing its importance for successful AI initiatives.
- Leverage AI governance services: Consider partnering with experts who can provide guidance on implementing the standard effectively within your specific organizational context.
The Future of Data Quality in Machine Learning
As AI and ML continue to evolve, data quality standards like ISO/IEC 5259-4 will play an increasingly critical role in ensuring responsible and effective implementation. The standard is expected to evolve alongside technological advancements, with updates and refinements based on implementation experiences and emerging best practices.
Organizations that invest in robust data quality processes today will be better positioned to leverage future AI innovations while maintaining trust and compliance. By establishing a solid foundation for data quality, ISO/IEC 5259-4 helps organizations build AI systems that are not only powerful but also reliable, fair, and trustworthy.
Practical Applications
ISO/IEC 5259-4 represents a significant milestone in the maturation of AI as a discipline, recognizing that high-quality data is fundamental to successful ML implementation. By providing a comprehensive framework for data quality processes across different ML methodologies, the standard helps organizations address one of the most critical challenges in AI development.
As organizations increasingly rely on AI and ML to drive innovation and competitive advantage, implementing robust data quality processes becomes not just a technical requirement but a strategic imperative. ISO/IEC 5259-4 offers a pathway to achieving this goal, enabling organizations to build AI systems that deliver reliable, accurate, and trustworthy results.
For organizations embarking on their AI journey or seeking to enhance existing initiatives, ISO/IEC 5259-4 provides a valuable roadmap for ensuring that their data foundation is solid. By investing in data quality today, organizations can unlock the full potential of AI while mitigating risks and building stakeholder trust.
Lorem ipsum dolor sit amet
Lorem Ipsum Dolor Sit Amet
Lorem ipsum odor amet, consectetuer adipiscing elit. Elementum condimentum lectus potenti eu duis magna natoque. Vivamus taciti dictumst habitasse egestas tincidunt. In vitae sollicitudin imperdiet dictumst magna.
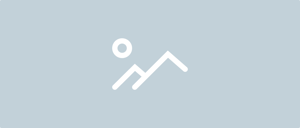
Lorem Ipsum Dolor Sit Amet
Lorem ipsum odor amet, consectetuer adipiscing elit. Elementum condimentum lectus potenti eu duis magna natoque. Vivamus taciti dictumst habitasse egestas tincidunt. In vitae sollicitudin imperdiet dictumst magna.
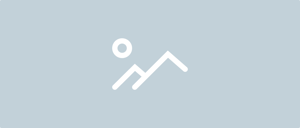
Lorem Ipsum Dolor Sit Amet
Lorem ipsum odor amet, consectetuer adipiscing elit. Elementum condimentum lectus potenti eu duis magna natoque. Vivamus taciti dictumst habitasse egestas tincidunt. In vitae sollicitudin imperdiet dictumst magna.
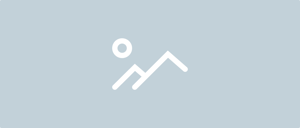
Lorem Ipsum Dolor Sit Amet
ISO/IEC Certification Support
Drive innovation and build trust in your AI systems with ISO/IEC certifications. Nemko Digital supports your certification goals across ISO/IEC frameworks, including ISO 42001, to help you scale AI responsibly and effectively.
Contact Us