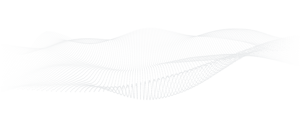
ISO/IEC TR 24027
This standard provides methods for assessing and mitigating bias within AI systems.
ISO/IEC TR 24027 describes a framework for addressing bias-related vulnerabilities throughout all phases of the AI system lifecycle, helping organizations to make their AI-aided decision-making equitable and trustworthy.
How does ISO/IEC TR 24027 identify and mitigate bias?
As our society becomes increasingly dependent on AI systems for governmental, commercial, and social relations, it is key to take a proactive approach to the bias that can become embedded in such systems. With the aid of ISO/IEC TR 24027, organizations can understand how bias arises and what measures can be taken to reduce its effects
ISO/IEC TR 24027 provides a definition of three major types of bias:
- Human cognitive biases can skew design choices, data collection procedures, and model training stages. Confirmation bias encourages individuals to interpret new information as evidence of their own beliefs. Automation bias may cause individuals to place too much trust in AI-aided decision making and other automated reasoning procedures.
- Data bias can emerge in even the most seemingly neutral of datasets. Underrepresentation or skewed sampling can occur in the data selection process as a result of immediate human cognitive biases or due to historical and systemic factors that continue to have impact on contemporary digital tools.
- Engineering bias can cause system behavior to become skewed towards certain outcomes over others. Biased system behavior can occur due to design choices, such as feature selection or algorithm tuning.
The primary method for combating bias is to assess datasets using metrics that evaluate how the model performs across different demographic groups. Various statistical formulas can be used to measure if the model exhibits unequal treatment based on sensitive attributes such as race, gender, age, or other individual characteristics. Key metrics include:
- Demographic parity, which measures if a decision-making system is equally likely to assign a positive outcome (e.g., approving a loan, granting parole) to different groups.
- Equalized odds, which prioritizes equal opportunity across demographics by requiring that a classifier should have equal false positive and false negative probabilities across different groups.
- Thematic fairness, which analyses whether an AI system would have made an alternative decision if an individual’s sensitive attributes had been different, given that all other factors stay constant.
However, bias mitigation is not a one-time effort. The employment of these metrics should be accompanied by a long-term plan as bias concerns can come up during all phases of the AI lifecycle. Accordingly, ISO/IEC TR 24027 emphasizes consistent monitoring, auditing, and transparent communications in order to make bias mitigation effective. Adopting the life-cycle approach that ISO/IEC TR 24027 recommends can keep your organization’s systems fair, effective, and socially forward.