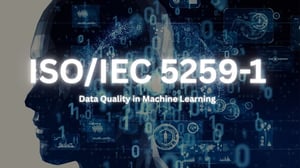
ISO IEC 5259-1: Key to Data Quality in Machine Learning
A standard for data quality in machine learning (ML)
Explore ISO/IEC 5259-1, the international standard for data quality in AI that helps organizations improve model accuracy and meet regulatory requirements
The quality of data directly impacts the reliability and effectiveness of artificial intelligence systems. ISO/IEC 5259-1 establishes the essential framework for ensuring data quality in analytics and machine learning applications, providing organizations with the tools needed to build trustworthy AI systems. This foundational standard sets the stage for implementing robust data quality practices throughout the AI lifecycle.
What is ISO/IEC 5259-1?

ISO/IEC 5259-1 serves as the cornerstone of the ISO/IEC 5259 series, focusing specifically on data quality for analytics and machine learning (ML). Published in 2024, this international standard provides a comprehensive overview, terminology, and illustrative examples to help organizations understand and implement effective data quality practices in AI systems.
As the foundation for conceptual understanding of data quality in AI applications, ISO/IEC 5259-1 establishes a framework for assessing and enhancing data quality across different phases of the data lifecycle. This framework is crucial for ensuring reliable analytics and ML outcomes in an increasingly AI-dependent business environment driven by information technology.
Why Data Quality Matters for AI Systems
The adage "garbage in, garbage out" is particularly relevant for AI systems. Even the most sophisticated machine learning algorithms cannot compensate for poor-quality data. According to research from MIT Sloan Management Review, organizations lose an average of 15-25% of their revenue due to poor data quality.
Key reasons why data quality is critical for AI systems include:
- Model Accuracy: High-quality data leads to more accurate predictions and insights in artificial intelligence applications.
- Reduced Bias: Properly vetted data helps minimize algorithmic bias and discrimination.
- Regulatory Compliance: Meeting data quality standards helps satisfy EU AI Act and other regulatory requirements.
- Resource Efficiency: Better data quality reduces computational resources needed for data cleaning.
- Trust and Adoption: Reliable AI outputs build stakeholder confidence and drive adoption.
The ISO/IEC 5259 Series: A Comprehensive Approach

ISO/IEC 5259-1 is part of a broader series of standards designed to address various aspects of data quality in AI and machine learning:
- ISO/IEC 5259-1: Overview, terminology, and examples (the foundation).
- ISO/IEC 5259-2: Data quality measures and models.
- ISO/IEC 5259-3: Data quality management requirements and guidelines.
- ISO/IEC 5259-4: Data quality process framework.
- ISO/IEC 5259-5: Data quality governance framework.
Together, these standards provide a comprehensive approach to managing data quality throughout the AI lifecycle, from data acquisition to model deployment and monitoring, ensuring high data quality at every step.
Key Components
Conceptual Framework
ISO/IEC 5259-1 establishes the conceptual framework for understanding data quality in the context of AI and machine learning. This includes defining the relationship between data quality and AI system performance, identifying key stakeholders, and outlining the scope of data quality considerations.
Terminology and Definitions
The standard provides a common language for discussing data quality in AI applications, ensuring that all stakeholders—from data scientists to business leaders—share a consistent understanding of key concepts. This shared vocabulary is essential for effective communication and collaboration across disciplines, making definitions clear for all users.
Illustrative Examples and Use Cases
To bridge theory and practice, ISO/IEC 5259-1 includes real-world examples and use cases that demonstrate how data quality impacts AI systems across various industries and applications. These examples help organizations understand the practical implications of data quality issues and how to address them effectively.
Data Quality Characteristics for AI
Building on the ISO/IEC 25012 data quality model, ISO/IEC 5259-1 identifies several key data quality characteristics particularly relevant for AI and machine learning:
Inherent Data Quality Characteristics
- Accuracy: The degree to which data correctly represents real-world values.
- Completeness: Whether all required data is present and available.
- Consistency: Freedom from contradictions within the dataset.
- Credibility: The trustworthiness of data sources and values.
- Currentness: How up-to-date the data is relative to the task.
System-Dependent Characteristics
- Accessibility: Ease of retrieving and using the data on your website.
- Compliance: Adherence to standards, regulations, and policies.
- Confidentiality: Protection of sensitive information.
- Efficiency: Resource requirements for data processing.
- Precision: Level of detail and exactness in the data.
Implementing in the Organization
Adopting ISO/IEC 5259-1 requires a strategic approach that considers your organization's specific AI objectives and data environment. Here are key steps to successful implementation:
- Assessment: Evaluate your current data quality practices against the standard's framework.
- Gap Analysis: Identify areas where your data quality management falls short.
- Strategy Development: Create a roadmap for implementing improved data quality practices.
- Governance Structure: Establish clear roles and responsibilities for data quality management.
- Process Integration: Embed data quality considerations into your AI development lifecycle.
- Continuous Monitoring: Implement ongoing assessment of data quality metrics.
Organizations looking to enhance their AI governance capabilities will find ISO/IEC 5259-1 particularly valuable as a foundation for building trustworthy AI systems.
Benefits of Adopting
Implementing ISO/IEC 5259-1 offers numerous advantages for organizations developing or deploying AI systems:
- Improved AI Performance: Higher-quality data leads to more accurate and reliable AI models.
- Risk Reduction: Systematic data quality management reduces the risk of AI failures and biases.
- Standardized Approach: A consistent framework for assessing and managing data quality.
- Enhanced Collaboration: Common terminology facilitates communication across teams.
- Regulatory Readiness: Alignment with emerging global AI regulations.
- Competitive Advantage: Transparency in AI becomes a market differentiator.
According to the National Institute of Standards and Technology (NIST), organizations that implement robust data quality frameworks are better positioned to manage AI risks and build trustworthy systems.
How ISO/IEC 5259-1 Relates to Other Standards
ISO/IEC 5259-1 doesn't exist in isolation but complements other important standards in the AI and data quality ecosystem:
- ISO/IEC 42001: AI management systems.
- ISO/IEC 23053: Framework for artificial intelligence systems using machine learning.
- ISO/IEC 38507: Governance implications of AI for organizations.
- ISO/IEC 24368: Overview of ethical and societal concerns.
Organizations pursuing comprehensive AI regulatory compliance should consider how these standards work together to address different aspects of responsible AI development and deployment.
Maximizing AI Value Through Quality Data
ISO/IEC 5259-1 provides the essential foundation for ensuring data quality in AI and machine learning applications. By establishing a common framework, terminology, and conceptual understanding, this standard enables organizations to implement effective data quality practices that lead to more reliable, trustworthy, and valuable AI systems.
To begin your journey toward improved data quality for AI:
- Familiarize yourself with the complete ISO/IEC 5259 series.
- Assess your current data quality management practices.
- Develop a strategic plan for implementing the standard's recommendations.
- Consider how data quality fits into your broader AI maturity and compliance efforts.
- Engage with experts who can guide your implementation process.
Ready to enhance your organization's approach to data quality in AI? Contact our team of experts today to learn how we can help you implement ISO/IEC 5259-1 and build more trustworthy AI systems that deliver real business value.
Lorem ipsum dolor sit amet
Lorem Ipsum Dolor Sit Amet
Lorem ipsum odor amet, consectetuer adipiscing elit. Elementum condimentum lectus potenti eu duis magna natoque. Vivamus taciti dictumst habitasse egestas tincidunt. In vitae sollicitudin imperdiet dictumst magna.
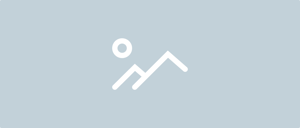
Lorem Ipsum Dolor Sit Amet
Lorem ipsum odor amet, consectetuer adipiscing elit. Elementum condimentum lectus potenti eu duis magna natoque. Vivamus taciti dictumst habitasse egestas tincidunt. In vitae sollicitudin imperdiet dictumst magna.
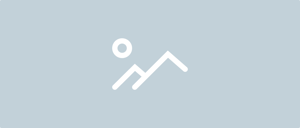
Lorem Ipsum Dolor Sit Amet
Lorem ipsum odor amet, consectetuer adipiscing elit. Elementum condimentum lectus potenti eu duis magna natoque. Vivamus taciti dictumst habitasse egestas tincidunt. In vitae sollicitudin imperdiet dictumst magna.
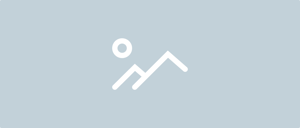
Lorem Ipsum Dolor Sit Amet
ISO/IEC Certification Support
Drive innovation and build trust in your AI systems with ISO/IEC certifications. Nemko Digital supports your certification goals across ISO/IEC frameworks, including ISO 42001, to help you scale AI responsibly and effectively.
Contact Us