In this article, we dive into the AI lifecycle and its role in governance, exploring how ethical, compliant, and efficient AI management integrates across all stages, referencing NIST’s Risk Management Framework.
The AI Lifecycle and its role in AI Governance
Managing the AI lifecycle and governance of your AI systems streamlines regulatory compliance and improves accuracy and performance. This improves operational efficiency by enabling early risk detection and ensuring optimal resource allocation. Organizations can achieve cost savings through efficient risk and resource management while continuously refining the quality of AI systems for better outcomes. Lastly, managing the lifecycle improves scalability, speeds up deployments and contract processes, and provides better business insights for better decisions.
NIST Risk Management Framework (RMF) 1.0 states that "AI governance functions may be performed in any order across the AI lifecycle," emphasizing the adaptability required for governance in diverse contexts. The lifecycle is not a rigid sequence but rather a flexible framework that organizations can adapt to meet their specific needs. Functions like risk assessment, policy updates, or compliance checks can occur at any stage, ensuring that governance is seamlessly integrated throughout the process.
Moreover, "the process should be iterative, with cross-referencing between functions as necessary." – NIST RMF 1.0. This means that governance is a continuous process where feedback loops are critical. For example, insights from the monitoring phase can inform re-evaluation and design improvements, while deployment outcomes can highlight areas for enhanced compliance. Cross-referencing ensures that all components of governance are interconnected, driving a holistic approach that adapts to changes in technology, regulations, and organizational priorities.
The AI Governance Lifecycle consists of interconnected stages that collectively ensure ethical and transparent AI management:
Inception: The first stage in the AI development lifecycle begins when stakeholders decide to transform an idea into a real system. It establishes a strategic foundation for AI projects, including goal setting and ethical considerations.
Design and Development: This stage creates the AI system and concludes with an AI system that is ready for verification and validation. Here, stakeholders ensure the AI system fulfils the original objectives, requirements and other targets identified during the inception stage.
Verification and Validation: Verification and validation checks that the AI system from the design and development stage works according to requirements and meets objectives.
Deployment: The AI system is installed, released or configured for operation in a target environment.
Operation and monitoring: The AI system is operational and monitored while in use. However, there is continuous tracking of the AI performance, incident reporting, and subjected to updates to meet new requirements to improve performance and reliability.
Re-evaluation: Periodically reviewing systems to align with evolving standards, regulations, and organizational objectives.
Retirement: Eventually, the AI system may become outdated, requiring repairs and updates that can't keep up with emerging needs. Thus processes such as decommissioning, discarding, and replacement may take place.
We have designed Nemko Digital's core AI governance services around key elements that can be integrated and iteratively used along the AI lifecycle. These elements are:
These elements help your organization manage both the AI system and internal processes. They allow you to Ensure organizational policies are aligned with global AI regulations and ethical best practices; define and update them as needed, 2) Perform risk assessments and implement mitigating strategies for AI operations, 3) Implement, among other things, the necessary tools for regulatory reporting and documentation.
Learn more about our AI governance services.
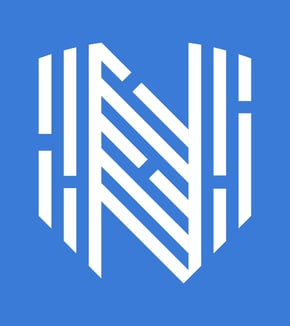